autoPET
Machine learning for hybrid medical imaging.
Databases, Codes and Leaderboards.
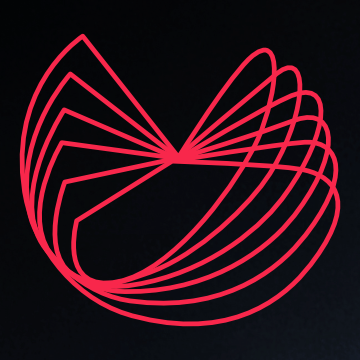
Machine learning challenges for hybrid medical imaging
Positron Emission Tomography / Computed Tomography (PET/CT) and CT are an integral part of the diagnostic workup for various malignant solid tumor entities. Currently, response assessment for cancer treatment is performed manually by clinicians. Despite the highly time-consuming nature of this manual task, only unidimensional (diameter) evaluations of a subset of tumour lesions are used to assess tumour dynamics.
Automation of tumour detection and segmentation as well as longitudinal evaluation may enable faster and more comprehensive information and data extraction. However, automated solutions for this task are lacking. Artificial Intelligence (AI)-based approaches may be an appropriate way to address lesion detection and segmentation in whole-body hybrid imaging (PET/CT and CT) to compensate workload and time pressure.
The segmentation frontier
Automated lesion segmentation on whole-body Fluorodeoxyglucose (FDG) PET/ CT data. The specific difficulty of lesion segmentation in FDG-PET lies in the fact that not only tumor lesions but also healthy organs (e.g. the brain) can have significant FDG uptake; avoiding false positive segmentations can thus be difficult.
The generalization frontier
Focus towards evaluating algorithmic robustness across different environments. A robust behavior of the algorithms in terms of moderate changes in acquisition protocol, acquisition site or PET tracers is needed.
The tracer frontier
Achieve multitracer and multicenter generalization of automated lesion segmentation. Generalization with an increased focus of appropriate data processing pipelines (data-centric approach) and/or the usage of pre-trained, foundational models can be helpful.
The human frontier
Explore the impact of integrating prior human knowledge in the form of an interactive segmentation in two databases: whole-body PET/CT, and longitudinal CT. Appropriate data processing pipelines (data-centric approach) and/or the usage of pre-trained, foundational models are allowed.
Large publicly available databases, codes and models are provided to support research in this area